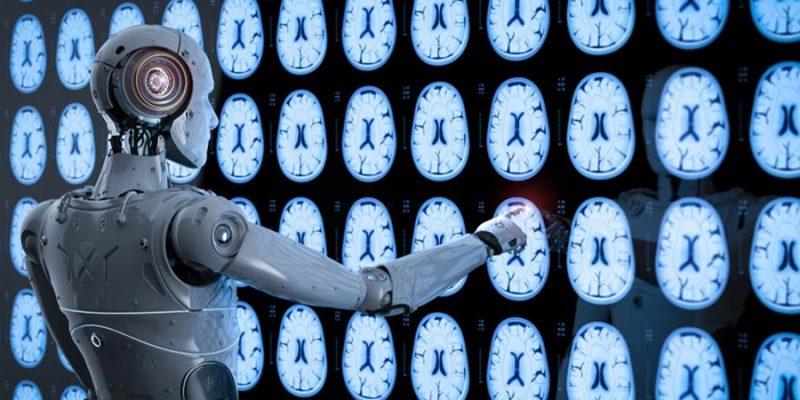
Here are some of the technologies that are making big moves in medical imaging AI
One of the components of research in the Medical Imaging service is the Product Development and Technology Trends file which considers some of the maximum impactful currents in medical imaging AI. The developments mentioned in the file are set to form the future of the AI marketplace in the upcoming years, especially as using machine learning in medical learning is at a pivotal point. While still a young sector, it is developing rapidly, with each technology maturing and medical implementations becoming clearer. Medical imaging AI solutions are turning into a better priority for both companies and vendors alike, that’s permitting some AI outfits to strike increasingly massive deals and set up leadership positions. Facilitating those changes are numerous key elements of medical imaging AI development.
The Transition Away from End Solutions
In its contemporary nascent state, the medical imaging AI marketplace is characterized by its fragmentation. It is made up of a host of unproven start-ups that have tended towards growing narrow AI tools that deal with a specific problem. However, radiologists still want to review an image to perceive different potential findings, limiting the benefits provided through point solutions, and we’ve got started to see these narrow AI algorithms evolving to comprehensive answers, end-to-end solutions, and AI suites. However, there remains a huge marketplace opportunity for narrow AI solutions addressing excessive value use cases, for example, fractional flow reserve computed tomography (FFR-CT), breast lesion detection, or C-spine fracture detection. The most notable of these exceptions is FFR-CT, in which a solitary measurement is all this is required. The specificity of its use is irrelevant given its capacity to enhance a patient’s care pathway and spare them from an invasive catheterization procedure. Comprehensive answers, locate extra numbers of findings for a given body area, goal to choose incidental or secondary findings, lowering the probability of missed diagnoses. They also can perceive different non-pathological findings (along with tube placements or anatomical positioning), eliminating the need for repetitive, a couple of scans in sure instances.
The Rise of Risk-based Screening
Risk-based screening is about to become greater widely applied in the coming years. At present, screening applications are frequently an effective, albeit relatively blunt tool. Risk-based screening applications will permit those maximum likely to be affected by a particular circumstance to be focused on and determined more closely. Those at less risk, on the alternative hand, might be spared unnecessary exams, permitting them to keep away from extra radiation exposure, in addition to different negative consequences, which includes unneeded biopsies, for example. This stratified method of screening may also keep providers money, permitting them to focus their assets on those patients most likely to require them.
AI Marketplaces are Becoming Platform-oriented
One of the obstacles to increased AI adoption in medical imaging AI is the closing mile challenges that healthcare companies face in enforcing answers into the workflow. These consist of elements like the choice and buying of applications, in addition to deployment, integration, and workflow orchestration. AI marketplaces coupled with AI platforms have addressed those demanding situations. AI marketplaces furnish companies with smooth get entry to a whole host of algorithms from a huge variety of carriers (selection and purchase of AI answers), with billing and contracting for all algorithms usually managed by the marketplace. While primarily targeted diagnostic equipment, those marketplaces’ services had been evolving to higher match the desires of companies, with numerous vendors increasing their marketplaces to consist of operational and analytics equipment along with their image analysis solutions.
A Strong Structure to Build On
The key to machine learning’s achievement in any field is the statistics that gear has at its disposal. In medical imaging AI, these foundational statistics will enjoy the growing prominence of established reporting. The ability to transform AI findings and free-textual content reports into a structured record containing each statistic and records the findings of interest might be key to maximizing the utility of medical imaging AI statistics. We have begun out with peer-based reporting gear input the market (for example, Smart Reporting and Nuance), however, those services are particularly constrained and continue to be in the early levels of development. AI developers may also consider releasing products themselves, even though given the extent of understanding and the specified resource; their time can be higher spent growing their core analysis products. Instead, partnership with a based reporting expert may also show to be a higher strategy long term.
Product Developments
Product developments are influencing the boom and evolution of the medical imaging AI market, and numerous key regions have been highlighted above. Narrow AI algorithms that address excessive value use cases (for example, FFR-CT) will stay viable offerings, however, carriers with AI algorithms need to don’t forget to evolve their products to complete or end-to-end solutions, relying on the use case. Imaging IT vendors may also appear to bundle native capabilities alongside third-party algorithms as AI suites or workflow packages.