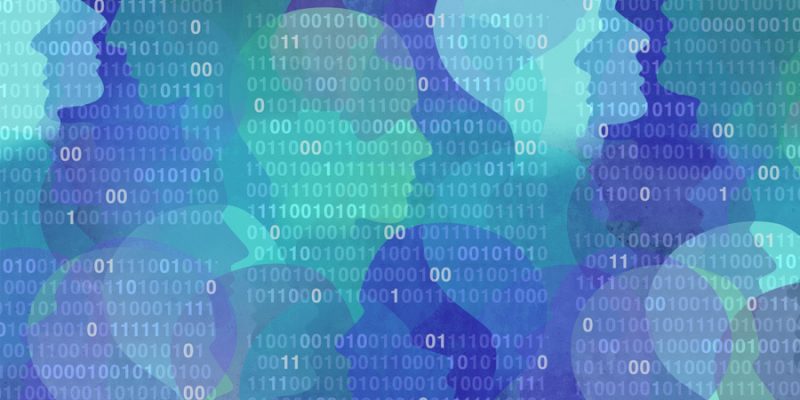
While AI brings improvements and blessings to medicine, it can additionally play a position in perpetuating racial bias in healthcare
The position of artificial intelligence is developing in healthcare, but many patients don’t have any concept their statistics are getting into contact with algorithms as they circulate through doctor appointments and scientific procedures. While AI brings improvements and blessings to medicine, it can additionally play a position in perpetuating racial bias in healthcare, now and then unbeknownst to the practitioners who depend on it. Journalists must take a nuanced method to report about AI toolsto unearth inequity, highlight advantageous contributions and inform patients’ memories in the context of broader studies. For perception on the way to cover the subject with nuance, The Journalist’s Resource spoke with HilkeSchellmann, an impartial reporter who covers how AI impacts our lives and a journalism professor at New York University, and Mona Sloane, a sociologist who research AI ethics at New York University’s Center for Responsible AI. Schellmann and Sloane have labored collectively on crossover initiatives at NYU, even though we spoke to them separately. This tip sheet is a partner piece to the studies roundup “Artificial intelligence can fuel racial bias in healthcare, however, can mitigate it, too.”
Keep your reporting socially and historically contextualized
Artificial intelligence can be a rising field, however, it intertwines with a deep-seated global inequality. In healthcare putting, in particular, racism abounds. For instance, research has proven health care experts routinely downplay and under-deal with the bodily ache of Black patients. In numerous fields such as dermatology, there’s also a loss of studies on human beings of color. Journalists masking synthetic intelligence need to explain such gear within “the lengthy and painful arc of racial discrimination in society and healthcare specifically,” says Sloane.
Collaborate with researchers critical that journalists and academic researchers
Deliver their relative strengths collectively to shed mild on how algorithms can work to both identify racial bias in healthcare and additionally to perpetuate it. Schellmann sees those agencies of human beings as bringing precise strengths to the table that make for “a mutually exciting collaboration.” Researchers tend to do their paintings on a good deal longer time limits than journalists, and inside instructional institutions, researchers regularly have to get entry to large quantities of facts than many journalists.
Consistent evaluation
Stanford University researchers highlighted in a perspective paper that demanding situations that result in bias and disparity in biomedical AI are carefully connected to the facts series and assessment of algorithms. To make certain the dependable and honest use of AI in fitness care, they endorse the regular assessment and monitoring of those tools; in addition to the collection of extra diverse facts.
Place patient narratives at the core of journalistic storytelling
In addition to the usage of peer-reviewed studies on racial bias in healthcare AI, or a journalist’s very own original research right into a company’s tool, its additionally essential newshounds encompass patient anecdotes. “Journalists want to speak to those who are affected by AI systems, who get enrolled into them without always consenting,” says Schellmann. But getting the stability proper among actual tales and skewed outliers is essential.
Stay skeptical
When private groups debut new healthcare AI tools, their advertising tends to rely upon validation research that tests their information’s reliability in opposition to an industry gold standard. Such research can appear compelling at the surface, however, Schellmann says journalists must stay skeptical of them. Look at a device’s accuracy, she advises. It must be 90% to 100%. These numbers come from an inner dataset that a company examines a tool on, so “if the accuracy is very, very low at the dataset that an organization built the set of rules on, that’s a big red flag,” she says.
Explain the jargon, and wade into the complexity
For beat journalists who frequently cover artificial intelligence, it can experience as though readers have to apprehend the basics. But it’s higher to assume audiences aren’t getting into every story with years of previous knowledge. Pausing in the center of a characteristic or breaking information to briefly define phrases is important to sporting readers via the narrative. Doing that is mainly crucial for terms such as “artificial intelligence” that don’t have constant definitions.
Ensuring transparency, privacy, and regulatory oversight
Another essential factor to ensure fairness in AI decision-making is transparency. This can take the form of cross-checking the selections of the set of rules with the aid of using humans and vice versa. In this way, they can preserve every different accountability and assist mitigate bias. And to ensure such transparency, the accompanying assisting infrastructure desires to be present.
Adopting algorithmic hygiene
When it involves information collection, Dr. Sanjiv M. Narayan, a cardiologist, and AI expert, recommends effective “algorithmic hygiene” as one of the satisfactory practices to hold bias out of AI This entails know-how the numerous reasons for bias and making sure that schooling facts are a consultant as lots as possible. “No facts set can constitute the whole universe of options. Thus, it’s miles vital to discover the goal application and audience upfront, after which to tailor the schooling facts to that goal.
Applying debiasing methods
Consensus on a method to degree or even define equity has yet to be achieved, however, measures are taken to grow the extent of equity in AI predictions. For this, researchers rent debiasing strategies to help lessen or eliminate differences throughout groups or people in keeping with sensitive attributes. To help builders adapt their work’s current debiasing strategies, IBM created the open-supply AI Fairness 360 toolkit.
SDoH in the Healthcare sector
Algorithm bias is trouble affecting a healthcare system that already shows boundaries to healthcare equity, which include unequal trying out and treatment, bias in clinical studies and records, and institutional racism. Research records and clinical information are much less likely to represent various minority groups’ black patients’ records correctly and as they should be because of socioeconomic inequalities in healthcare delivery.